With the advent of AI/ML models in the last years, users have come to expect more and more advanced features from the services and applications that can be only efficiently solved by incorporating AI/ML approaches and techniques and integrating them into modern digital ecosystems.
However, it is crucial to recognize that the seamless incorporation of these intelligent models into our digital ecosystem requires robust traditional IT frameworks. Even as artificial intelligence reshapes the landscape of user interaction and data processing, the foundational IT systems — including database management, network infrastructure, software development life cycles, and system architectures — remain indispensable.
These systems and methodologies provide the necessary groundwork for AI based features to function effectively, ensuring scalability, security, compliance and ease of maintenance of such AI enabled systems.
Therefore, while organizations will and must innovate with AI/ML technologies, these innovations still must honor the principles of good software development practices.
One of the key challenges of introducing AI/ML models either into existing or greenfield IT systems is how to successfully integrate the training and inference components of the solution.
Every AI/ML solution, by definition, consists of two distinct components.
Training area
Inference area
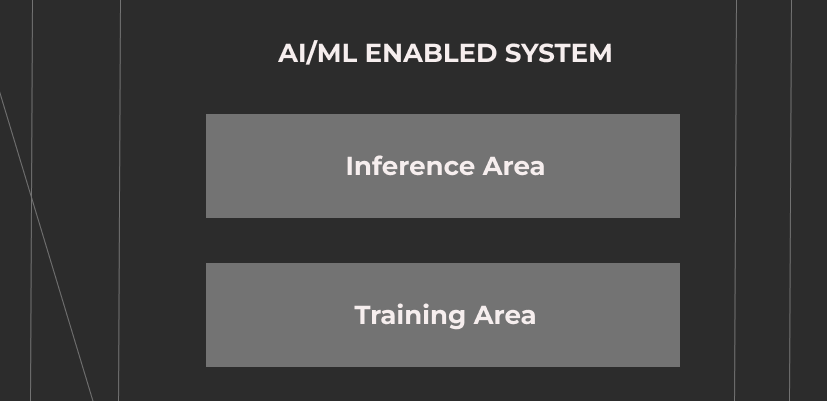
The purposes, computational, deployment and compliance requirements are different for each area. While there is a myriad of challenges to be solved in each area individually, the key to successful AI/ML initiatives will, at its core, be in coming up with the solutions that will complement both areas.
When organizations think about business requirements and AI/ML enabled features, the emphasis is always on the Inference area since this is the part of the solution that is visible to end users and customers. In most cases, the inference area becomes an integrated part of an already existing digital ecosystem. This is the area where AI/ML models live and are executed to provide some useful function to users.
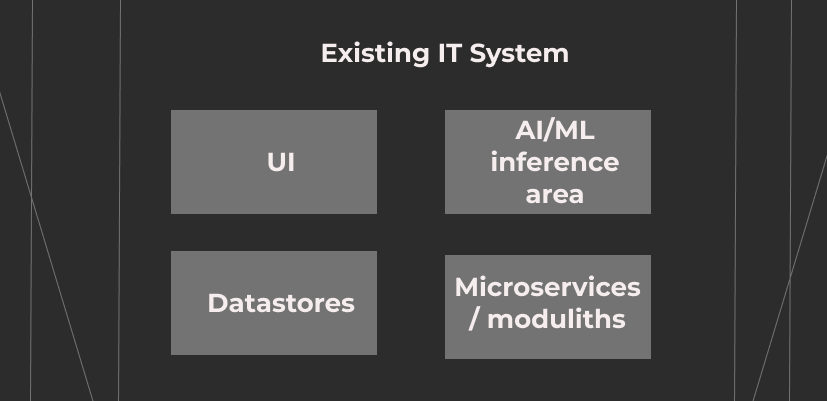
This means that successful realization of an AI/ML inference area and its integration must be done using tried and tested traditional solutions streamlined for speed and efficiency. e.g streaming platforms, distributed systems, cloud solutions, edge computing, microservices.
The Training area, on the other hand, must provide a sandbox environment for data scientists to perform experiments, model training, tuning and retraining. While inference is focused on speed and efficiency, the training area puts focus on running high-performance computing tasks often utilizing special compute hardware such as GPU and TPU. In most organizations these types of loads are new and there is no institutional knowledge and experience to leverage. This poses unique challenges when introducing AI/ML based workloads into an organization.
Architecting Digital Ecosystems
In summary, the integration of AI/ML into complex IT systems Is not just a matter of plugging in a new technology. It requires thoughtful re-architecting of the systems, where both the training and inference area are designed to fulfill their unique roles effectively.
Falls Sie Fragen haben, sind wir nur einen Klick entfernt.