Credit card fraud is a growing threat in the digital age, causing significant financial losses. Juniper Research predicts that the global cost of online payment fraud will reach $206 billion by 2025, up from $130 billion in 2020. This increase highlights the escalating threat. Traditional fraud detection methods, such as rule-based systems and blacklisting, struggle to keep pace with evolving fraud tactics.
Next-generation AI and MLOps offer robust solutions to these challenges. However, the effectiveness of these technologies hinges on robust AI governance. Ensuring AI compliance with regulations like the EU AI Act is crucial for building trustworthy AI systems in financial fraud prevention. AI governance frameworks help manage risks, provide transparency, and maintain ethical standards, making them essential in the fight against credit card fraud.
When MLOps meets AI governance for responsible AI
Nexi’s collaboration with CROZ exemplifies how setting up a state-of-the-art machine learning (ML) model for fraud detection requires strong MLOps practices. MLOps is essential for operationalizing responsible AI in financial fraud detection, ensuring that AI systems are developed, deployed, and maintained in a trustworthy and AI-compliant manner.
Key components of MLOps include version control for model iterations, which allows teams to track changes and improvements over time. Automated testing and validation are crucial for detecting fairness and bias, ensuring that models make equitable decisions. Continuous monitoring of model performance and drift detection helps maintain the accuracy and reliability of AI systems in dynamic environments.
While MLOps remains an essential set of principles for managing and organizing data and AI modeling, the evolution of the current regulatory framework has introduced AI governance.
AI governance is crucial for building trustworthy AI systems and ensuring compliance with regulations. AI governance complements the MLOps lifecycle by integrating the models’ technical monitoring into a regulatory framework and the relative business context. It provides the responsible development and use of AI, mitigating risks such as biases, discrimination, and privacy violations. By fostering trust and transparency in AI decision-making, governance frameworks promote innovation within a defined ethical framework.
AI Governance & Compliance in Financial Fraud Prevention
Several angles must be considered to understand the importance of AI governance and its connection to MLOps. In this blog, we examine Nexi, a European operator likely to be affected by the coming effects of the EU AI Act (more information is available at this link). Nonetheless, some of the processes and steps can be applied more generally to other jurisdictions and regulatory frameworks. Finally, additional regulations like GDPR and DORA are also highly relevant to the financial industry and shape the development of AI and ML systems.
The EU AI Act regulates the risk management of an AI solution that reaches users on the market. Organizations involved in the development should assess and manage those risks, like product safety measures.
Actors Defined in the EU AI Act
The EU AI Act outlines several key actors involved in the AI ecosystem. Let’s try to identify them for the finance industry.
- Providers are entities that develop AI systems to place on the market or put into service under their name or trademark. This could be a tech company specializing in AI development, a software company creating specific AI solutions for finance, or a large financial institution with in-house AI development capabilities.
- Users are any natural or legal persons using an AI system, such as banks, insurance companies, or investment firms implementing AI in their operations.
- Though less common in the financial industry, importers and distributors play roles in bringing AI systems into the EU market and making them accessible without altering their properties.
- While not typically applicable to finance, product manufacturers are also defined as those who integrate AI systems with their products under their own name or trademark.
Risk Classification and Examples of AI Use Cases for the Financial Industry
In the financial industry, the EU AI Act classifies AI use cases into risk categories, each with specific requirements and implications.
Unacceptable Risk (Prohibited)
🔴 Social Scoring: AI systems that create scores summarizing an individual’s social behavior and economic status for financial decisions are prohibited. Such practices could lead to discrimination and exclusion
🔴 Emotion Recognition/Manipulation: AI used to analyze or manipulate a person’s feelings for financial gain is banned. This could exploit vulnerabilities and pressure individuals into making unfavorable decisions
🔴 Real-Time Remote Biometric Identification: Unless highly regulated, systems using facial recognition or other biometrics for mass surveillance or identification in public spaces are prohibited due to their potential impact on privacy and freedom of movement
High Risk (Must Comply with Multiple Requirements and Undergo a Conformity Assessment)
🟡 Credit Scoring and Loan Approvals: AI systems determining creditworthiness and loan eligibility must be fair, unbiased, and explainable to avoid discriminatory practices
🟡 Fraud Detection and Risk Management: AI used to detect fraud or manage financial risk should be robust and accurate to prevent false positives and negative impacts on customers
🟡 Algorithmic Trading: AI-powered trading systems need safeguards to prevent market manipulation or instability
These high-risk AI use cases must adhere to strict requirements, including data quality and bias mitigation, transparency and explainability, human oversight and risk management, robustness and cybersecurity.
Lower Risk (Recommended to Apply High-Risk Practices and Subject to Transparency Obligations)
🟢 Chatbot
🟢 Deep Fakes
🟢 Speech Analytics
🟢 Customer Experience Improvement Systems
🟢 AI Systems for Customer Pattern Analysis
🟢 Money-Laundering Monitoring AI Systems
For example, in the case of Nexi, an anti-fraud solution is an AI use case, and it is evaluated to determine whether the EU AI Act applies. Since the solution is marketed in the EU, Nexi is classified as a provider. The anti-fraud solution is identified as a high-risk AI use case, and Nexi must derive and fulfill the relevant obligations to ensure compliance. In this scenario, CROZ is the BizTech company specializing in modeling AI for fraud detection and acts as the provider. Nexi, as the user, implements this AI system into their credit approval process.
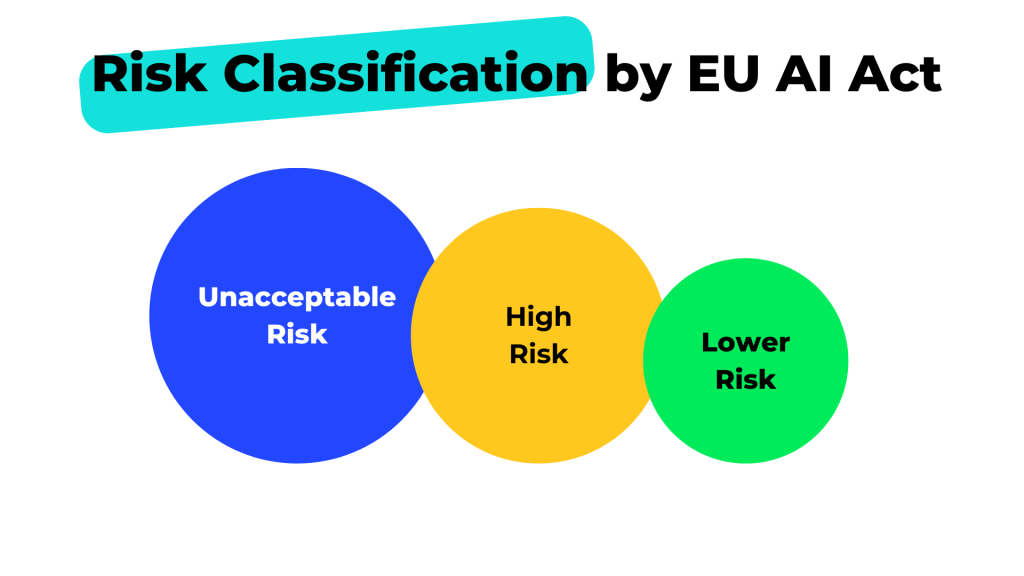
A step-by-step approach to AI governance
Defining and prioritizing AI use cases is iterative and involves several key steps (appliedAI, 2024). This framework ensures that AI governance is integrated and aligns regulations with business needs and technical scope.
Ideation: This step involves identifying AI use cases that align with the organization’s AI vision. For each use case, it is crucial to determine whether the EU AI Act is applicable and to classify the relevant risk category. This includes mapping obligations and defining roles, such as whether the organization acts as a developer or provider of the AI system.
Assessment: In this phase, the value and ease of implementation dimensions are assessed. From a governance perspective, this includes operationalizing AI governance by securing support and budget from high-level management, outlining responsibilities and human oversight, summarizing the status quo against legal obligations, creating technical documentation, and reviewing governance mechanisms to include affected stakeholders.
Prioritization: This step involves iteratively aligning the portfolio of use cases with key performance indicators (KPIs) for value and ease of implementation. This ensures that the most impactful and feasible use cases are prioritized.
Execution of Case in Production: During this phase, demonstrating compliance is critical, especially for high-risk use cases. Providers must undergo a conformity assessment before placing the solution on the market, and deployers of high-risk AI use cases have separate sets of obligations to fulfill.
Building a team for MLOps and AI governance
AI governance boosts documentation and monitoring, all aspects that thrive under the MLOps principles. This includes nesting AI use cases within governance and risk management frameworks, tracking AI assets across all project stages, and maintaining model risk lineage throughout the ML workflow.
Effective MLOps for responsible AI requires a collaborative effort from a diverse team with interdisciplinary competencies. For example, a team that manages AI governance and MLOps can cover the following roles.
- The model owner is responsible for risk management, ensuring the AI system adheres to regulatory requirements and mitigates potential risks.
- Data engineers handle the infrastructure, setting up and maintaining the data pipelines and environments necessary for model development and deployment.
- Model developers create and refine the AI models, focusing on achieving high performance and accuracy.
- Model validators rigorously test and validate the models to ensure they are fair, unbiased, and reliable.
- Model approvers review and approve the models before deployment, ensuring all governance and compliance standards are met.
- Finally, model deployers manage the deployment process, integrating the models into production environments and monitoring their performance.
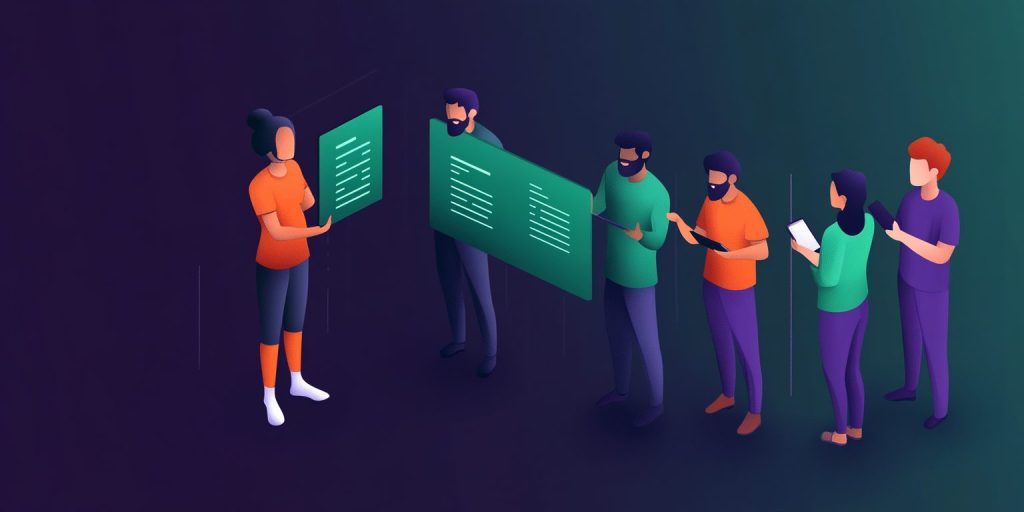
Hands-on: Designing and Deploying AI Governance
As we look ahead, it’s clear that the landscape of AI regulation is evolving. Implementing the EU AI Act requires standards that are still under development and subject to sector-specific rules in industries like fintech, healthcare, and automotive. Moreover, AI regulation is a global phenomenon. From 2022 to 2023, the number of mentions of AI in legislative proceedings nearly doubled, with discussions taking place in 49 countries, highlighting the global reach of AI policy discourse (source: The AI Index Report).
Designing and deploying an AI governance framework is the next step to fostering a culture of compliance and innovation, building trustworthy AI systems that benefit everyone. At CROZ, we embrace a looping paradigm for MLOps and AI governance, continuously refining our processes based on practical application and community insights.
Our journey begins with defining AI use cases and identifying key actors, focusing on risk identification and stakeholder engagement. We prioritize governance to ensure ethical AI use and integrate AI applications within a broader governance and risk management framework. Once governance structures are in place, we implement robust MLOps practices for model development and deployment. Check out our related blog page for a deeper dive and a technical demo on fraud detection!